Scientific Models: Essential Tools for Understanding Complex Systems
The foundation of scientific inquiry
Scientific models stand as one of the virtually powerful tools in the scientific arsenal. These representations of reality help scientists make sense of complex phenomena that might differently remain beyond our grasp. But what incisively make these models hence crucial to scientific progress?
At their core, scientific models are simplified versions of reality design to highlight specific aspects of natural phenomena. They serve as bridges between abstract theories and observable data, allow researchers to test hypotheses and make predictions about how systems behave under various conditions.
Types of scientific models
Scientists employ various types of models depend on their research questions and the phenomena they study:
Physical models
Physical models are tangible representations that mimic the structure or behavior of their subjects. The classic example is the DNA double helix model construct by Watson and crick, which help visualize the structure of this complex molecule. Other examples include scale models of the solar system, anatomical models of the human body, or wind tunnel models use to test aerodynamics.
These three-dimensional representations provide immediate visual and tactile understanding that might be difficult to grasp from equations or descriptions entirely. They’re especially valuable in educational settings where abstract concepts can be challenging to communicate.
Mathematical models
Mathematical models use equations and formulas to describe relationships between variables. These models excel at prediction and quantification, allow scientists to calculate expect outcomes under specific conditions.
From newton’s laws of motion to complex climate models with thousands of variables, mathematical models provide precision and predictive power. They enable scientists to express relationships in universal language that transcend cultural and linguistic barriers.
Conceptual models
Conceptual models represent ideas and relationships through diagrams, flowcharts, or mental frameworks. These models help organize think about complex systems without inevitably quantify every aspect.
Examples include food web diagrams in ecology, the periodic table in chemistry, or conceptual frameworks for understand disease transmission. These models emphasize relationships and interactions quite than precise measurements.
Computational models
With the advent of powerful computing technology, computational models have revolutionized science. These models use algorithms and computer simulations to represent complex systems and predict their behavior.
Weather forecasting models, protein fold simulations, and galaxy formation models all rely on computational approaches to tackle problems also complex for analytical solutions. These models can integrate vast datasets and run thousands of iterations to capture system dynamics.
The essential functions of scientific models
Scientific models serve multiple crucial functions in the advancement of knowledge:
Simplification of complex systems
Reality is messy and complicated. Models strip aside unnecessary details to focus on the elements about relevant to the question at hand. This simplification make complex systems manageable for study.
Consider climate models. The actual climate system involve countless interactions between atmosphere, oceans, land surfaces, and living organisms. A model might focus on key variables like greenhouse gas concentrations, solar radiation, and ocean circulation patterns while simplify or omit less critical factors.
Visualization of abstract concepts
Many scientific phenomena occur at scales or in dimensions that defy direct observation. Models translate these abstract concepts into forms we can visualize and comprehend.
Atomic models help us visualize particle overly small to see. Astronomical models represent cosmic structures span millions of light years. Statistical models visualize patterns in data that might differently remain hidden in numerical tables.
Prediction and hypothesis testing
Possibly the virtually powerful aspect of scientific models is their predictive capability. A good model doesn’t precisely describe what we already know — it predicts what we should observe under new conditions.
When these predictions match experimental results, they strengthen confidence in the underlying theory. When predictions fail, they highlight gaps in understanding and drive refinement of the model. This cycle of prediction, testing, and refinement form the backbone of scientific progress.
Communication and collaboration
Models provide a common language for scientists to communicate complex ideas. They serve as share reference points that facilitate collaboration across disciplines, institutions, and national boundaries.

Source: snapstudy.ai
When researchers publish models alongside their findings, they enable others to build upon their work, test alternative hypotheses, or apply the models to new contexts. This collaborative aspect accelerates scientific progress exponentially.
Limitations and evolution of scientific models
Despite their power, scientific models come with inherent limitations that wise researchers acknowledge and address:
All models are approximations
The famous statistician George box note that” all models are wrong, but some are useful. ” tThisfundamental truth remind us that models are invariably simplifications that capture some aspects of reality while miss others.
Recognize this limitation help scientists maintain appropriate skepticism and continue to refine their understanding. The virtually useful models clear define their boundaries and assumptions, allow users to judge when they might break down.
Models reflect current knowledge
Scientific models evolve as knowledge expand. The history of science is fill with examples of models that serve substantially for a time before being replaced by more comprehensive frameworks.
The geocentric model of the universe give way to the heliocentric model. The plum pudding model of the atom yield to the Bohr model and finally to quantum mechanical models. Each iteration incorporate new observations and address limitations of previous versions.
Balance simplicity and accuracy
Model builders face an inherent tension between simplicity and accuracy. Simple models are easier to understand and use but may miss important aspects of the system. Complex models might capture more details but become unwieldy and difficult to interpret.
The principle of parsimony, frequently call Occam’s razor, suggest that scientists should prefer the simplest model that adequately explain the observations. Find this balance require both technical skill and scientific judgment.
Case studies: models that change science
The Bohr model of the atom
When Niels Bohr propose his model of atomic structure in 1913, it revolutionizes our understanding of matter. By visualize electrons orbit the nucleus in discrete energy levels, the modelexplainsn the mysterious spectral lines observe in elements.
Though posterior supersede by quantum mechanical models, Bohr’s model remain valuable for teach basic atomic principles and demonstrate how level imperfect models can advance scientific understanding.
The double helix model of DNA
Watson and crick’s 1953 model of DNA structure stand as one of science’s most consequential models. By right represent the double helix arrangement, it instantly suggests how genetic information might be copy and transmit.
This model launch the modern era of molecular biology and demonstrate how a good structural model can generate insights far beyond its original purpose.
Climate models
Modern climate models integrate atmospheric physics, oceanography, and biogeochemistry into computational frameworks that simulate earth’s climate system. These models have proved unmistakably accurate in predict global temperature trends and have become essential tools for understand climate change.
Their evolution from simple one dimensional models to sophisticated global simulations illustrate how models grow more powerful as compute capabilities advance and scientific understanding deepens.

Source: elevate.in
Models in scientific education
Beyond research applications, models play a crucial role in science education:
Scaffolding complex concepts
Educational models provide conceptual scaffolding that help students build understanding increasingly. Teachers frequently begin with simplified models before introduce complexities and exceptions.
This approach acknowledge that learn, like science itself, frequently advance through progressively refine models sooner than immediate jumps to complete understanding.
Develop model literacy
Modern science education emphasize not simply memorize models but understand how models work as tools. Students learn to evaluate models critically, recognize their strengths, limitations, and domains of applicability.
This” model literacy ” repare students to engage with scientific information thoughtfully, whether they pursue careers in science or merely need to make informed decisions as citizens.
The future of scientific modeling
Scientific modeling continue to evolve with technological and methodological advances:
Integration of multiple models
Complex problems like climate change or disease outbreaks require integrate multiple specialized models. Researchers are developed frameworks that allow models from different disciplines to communicate and work unitedly, create more comprehensive understanding of interconnect systems.
Machine learning and AI in modeling
Artificial intelligence approaches are transformed scientific modeling by identify patterns in data overly complex for human analysis. These techniques can discover relationships that might not be apparent from first principles and help refine exist models.
From drug discovery to materials science, machine learning models are accelerated research and open new avenues for investigation.
Participatory modeling
Progressively, scientists recognize the value of incorporate diverse perspectives in model development. Participatory modeling bring unitedly researchers, stakeholders, and community members to build models that reflect multiple forms of knowledge and address real world needs.
This approach prove particularly valuable for environmental and social systems where human behavior and values play crucial roles.
Conclusion
Scientific models represent one of humanity’s virtually powerful intellectual tools. By create simplified representations of reality, they enable us to grasp complex phenomena, make testable predictions, and communicate sophisticated ideas.
The limitations of models remind us that science remain an ongoing journey sooner than a destination. Each model represent our best current understanding, invariably subject to refinement or replacement as new evidence emerge.
As science will tackle progressively complex challenges — from climate change to pandemic disease to artificial intelligence — models will continue to will serve as essential guides, will help us will navigate territory besides vast or complex to will comprehend direct. Understand how these models work, what they can tell us, and where they might mislead us remain a fundamental aspect of scientific literacy in the modern world.
This text was generated using a large language model, and select text has been reviewed and moderated for purposes such as readability.
MORE FROM searchhole.com
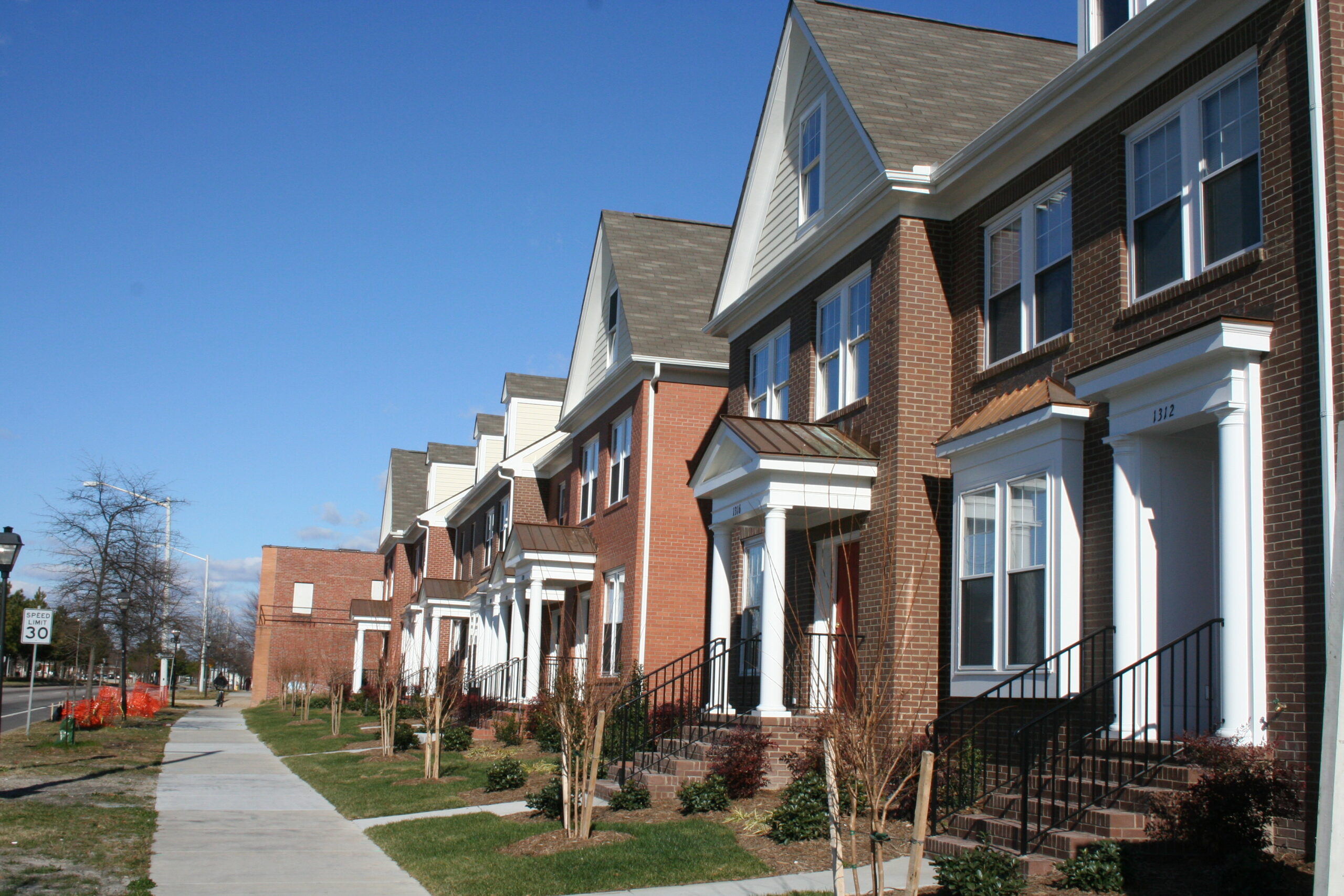
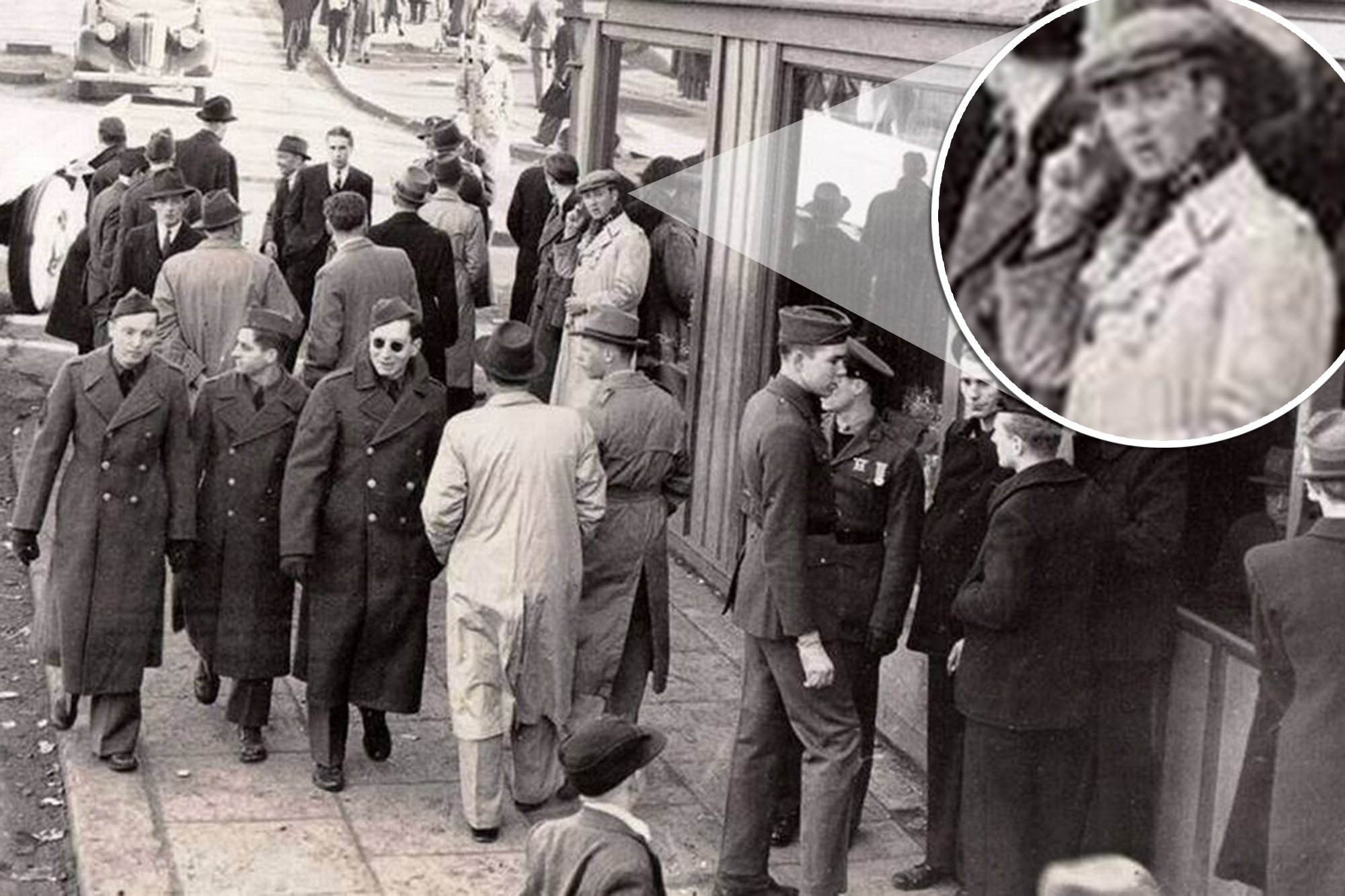
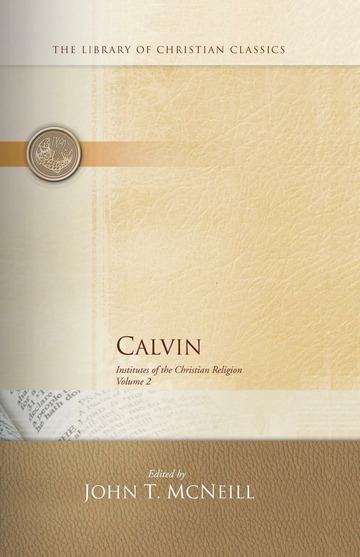
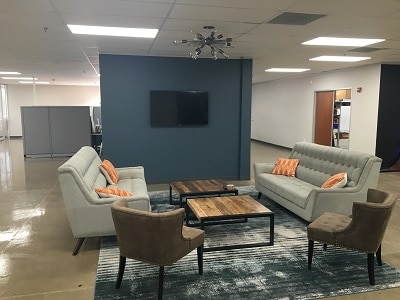
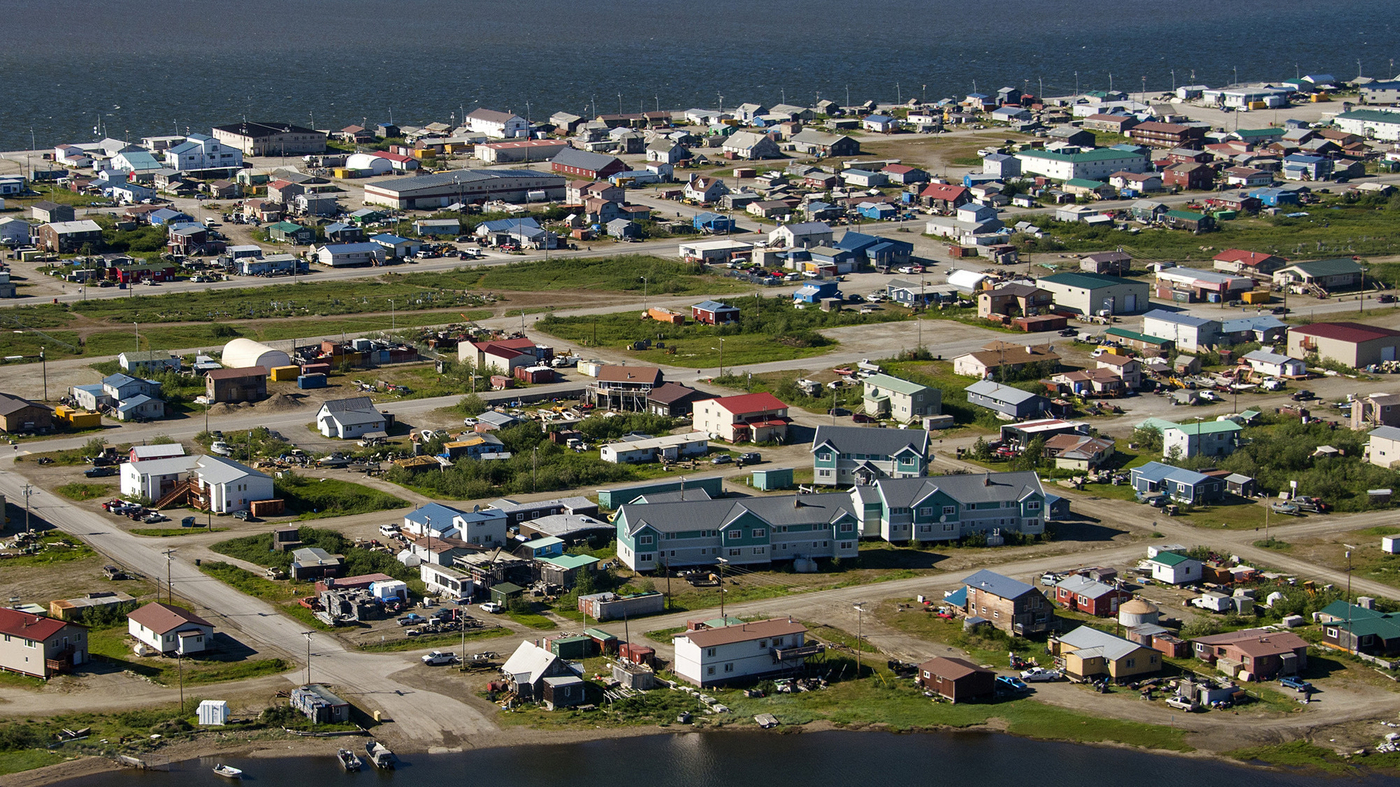
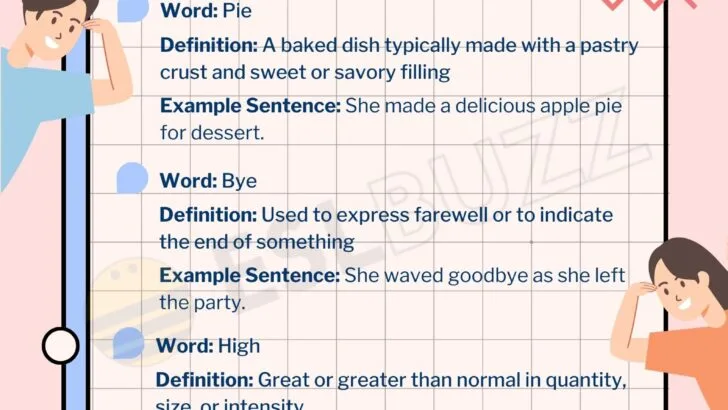
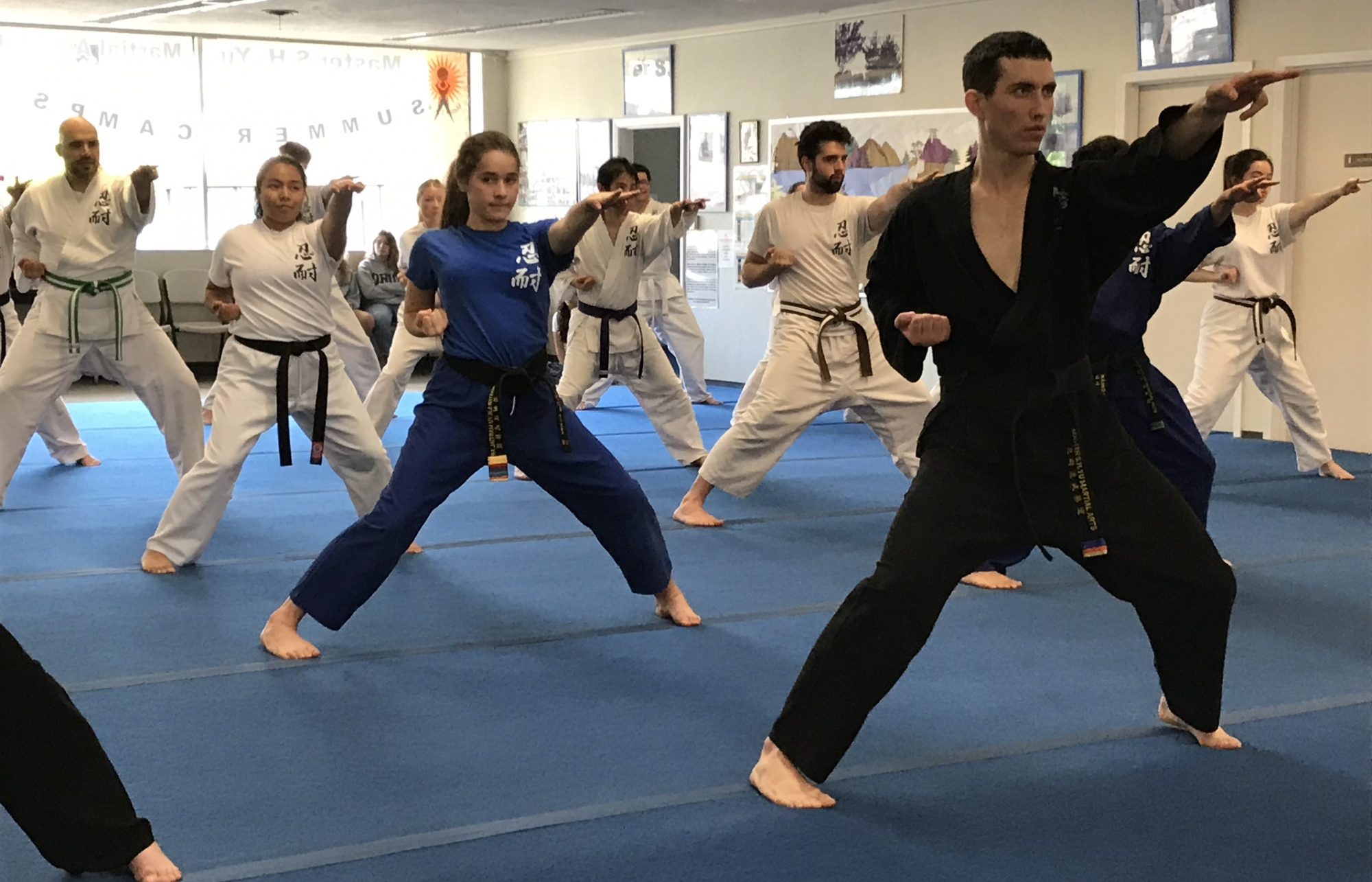
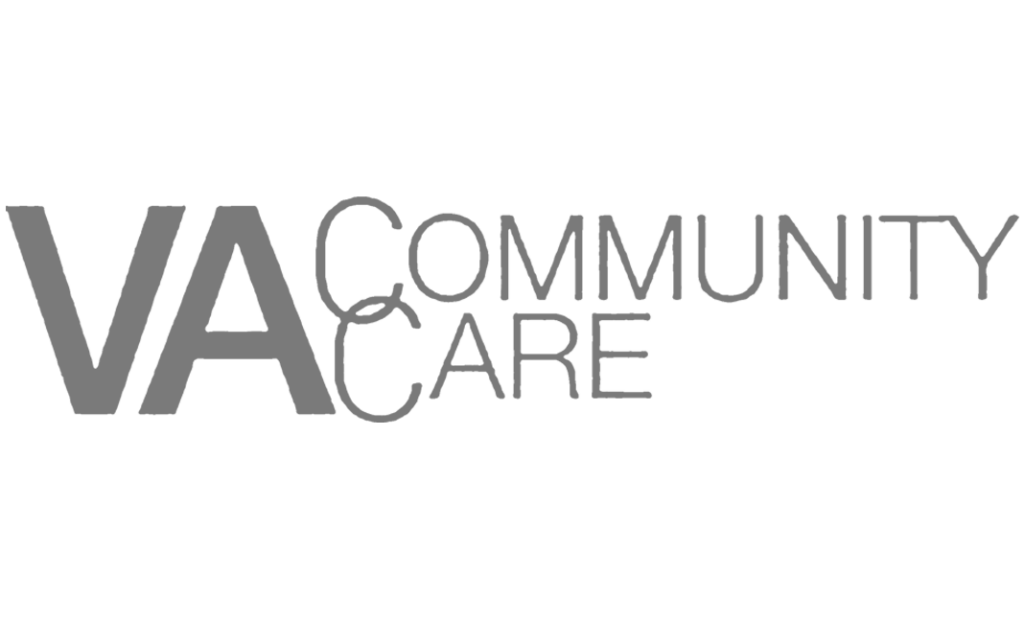
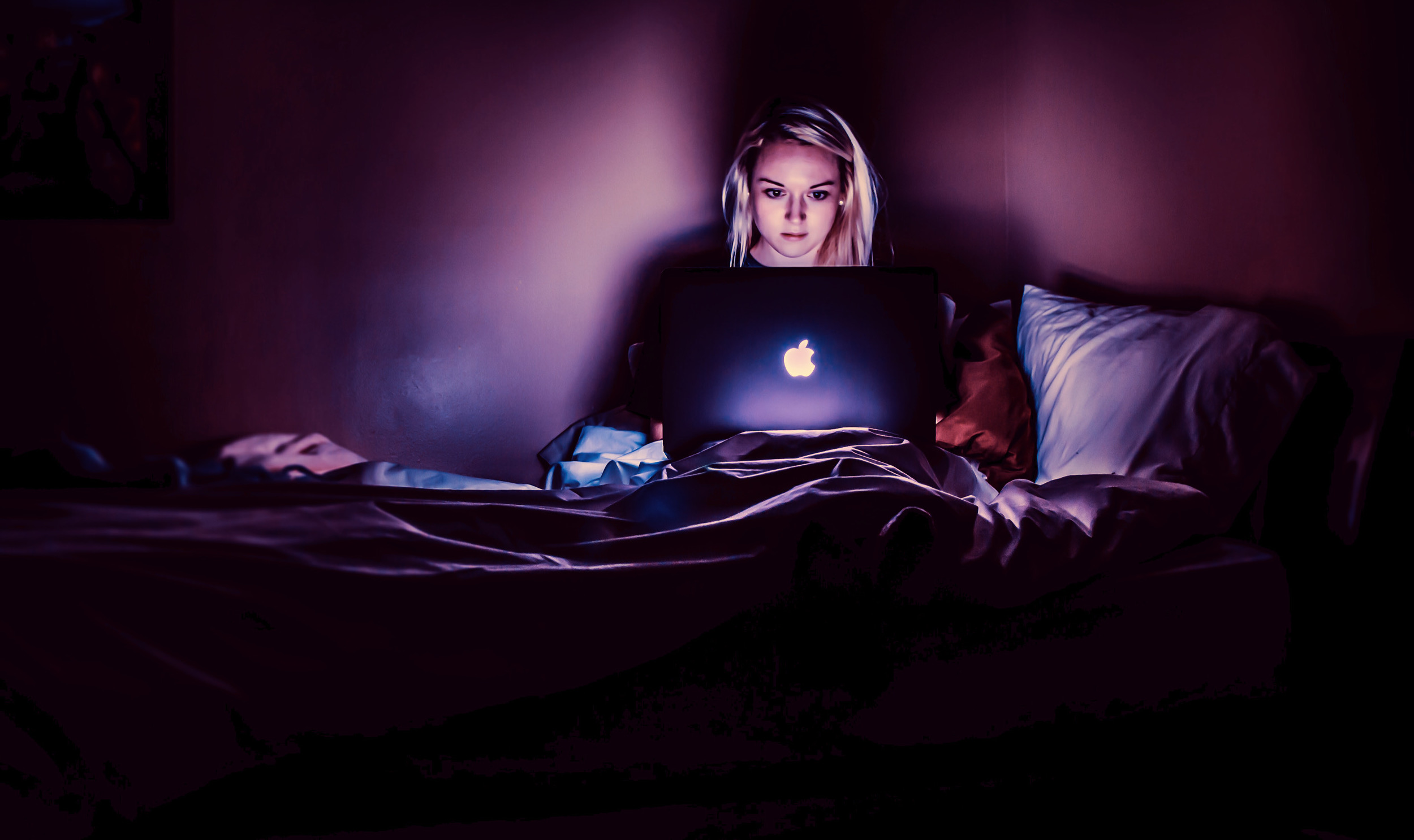
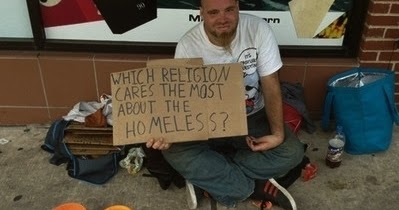
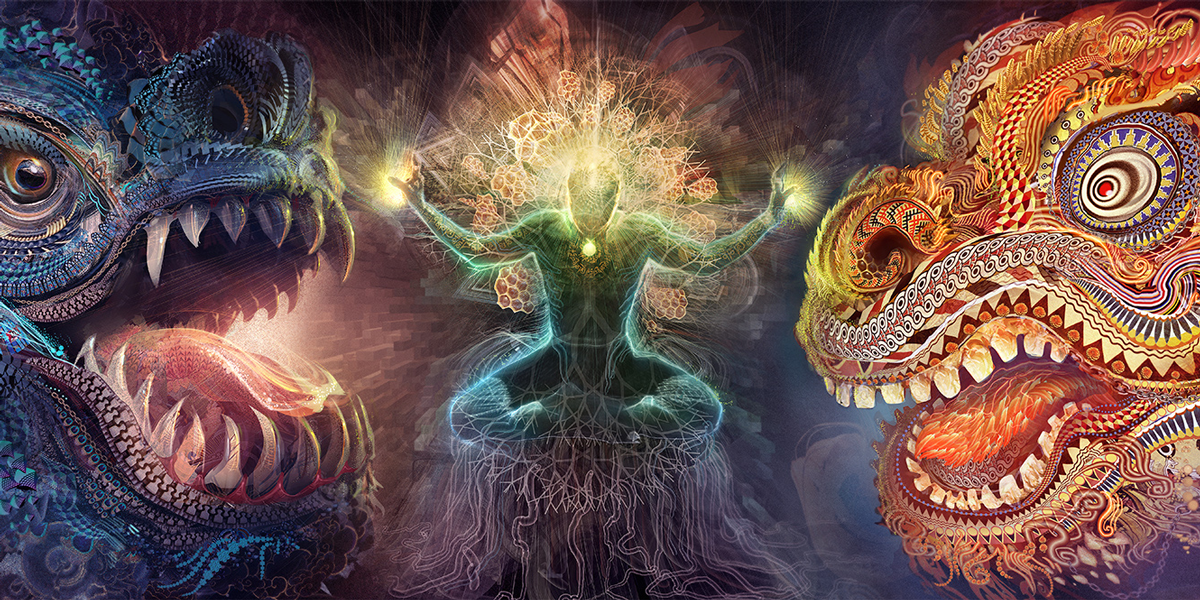
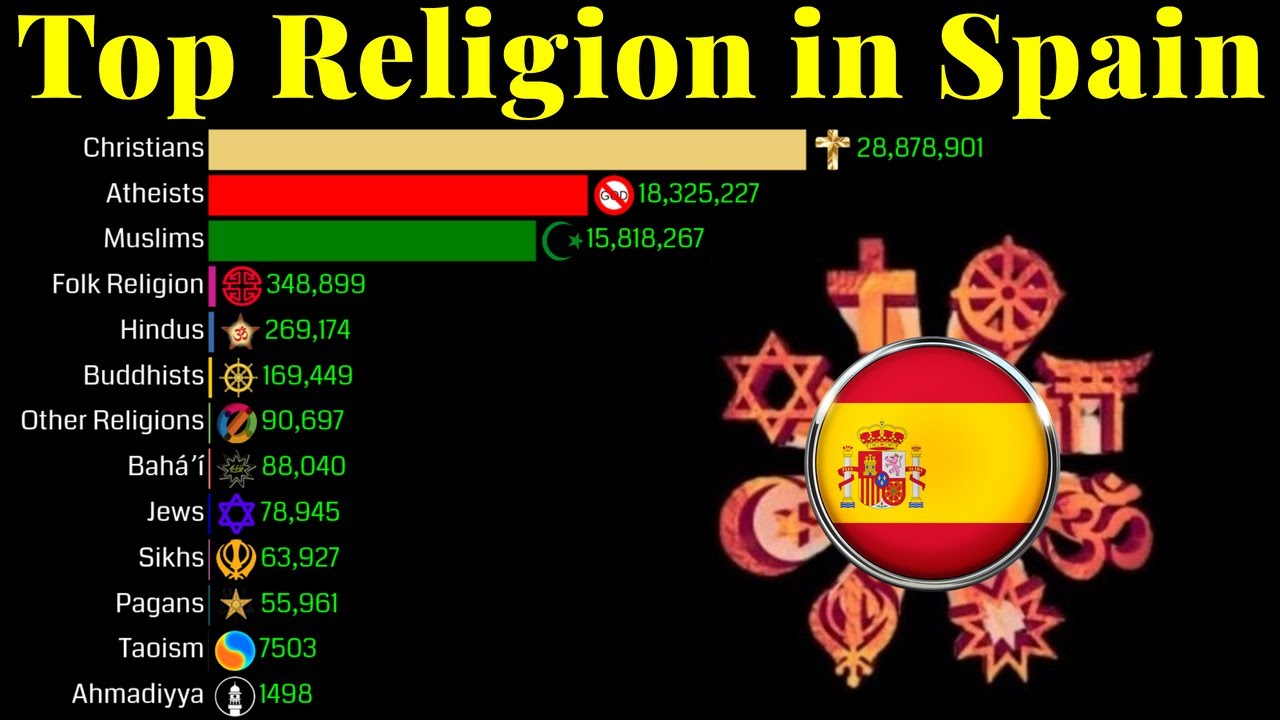